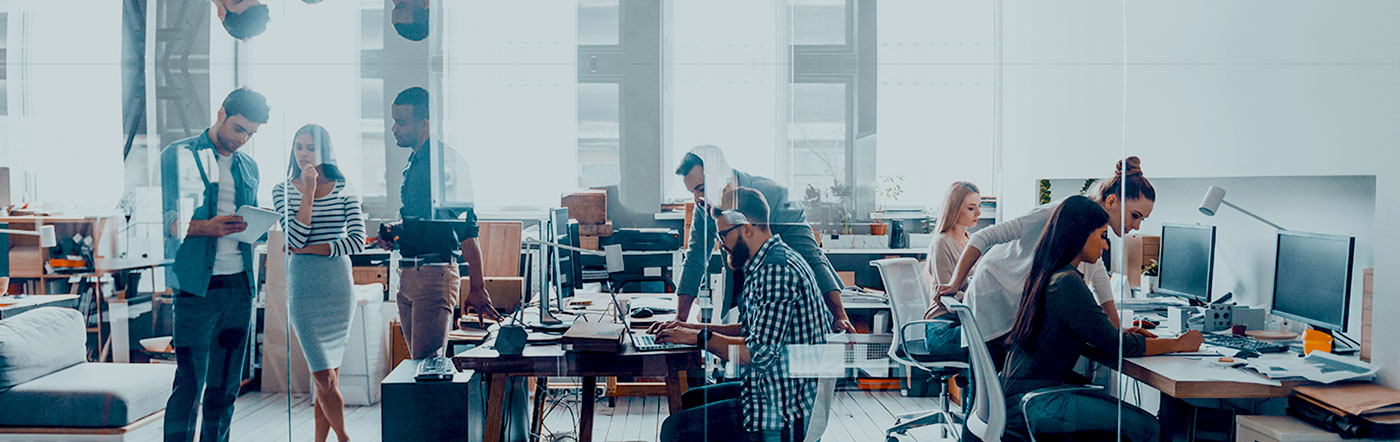
Company News
A Good Practice Towards Top Performance of Face Recognition: Transferred Deep Feature Fusion
|
Lin Xiong, Jayashree Karlekar, Jian Zhao, Jiashi Feng, Sugiri Pranata, Shengmei Shen
arXiv, 2017, 2017.04
Unconstrained face recognition performance evaluations have traditionally focused on Labeled Faces in the Wild (LFW) dataset for imagery and the YouTubeFaces (YTF) dataset for videos in the last couple of years. Spectacular progress in this field has resulted in saturation on verification and identification accuracies for those benchmark datasets. In this paper, we propose a unified learning framework named Transferred Deep Feature Fusion (TDFF) targeting at the new IARPA Janus Benchmark A (IJB-A) face recognition dataset released by NIST face challenge. The IJB-A dataset includes real-world unconstrained faces from 500 subjects with full pose and illumination variations which are much harder than the LFW and YTF datasets. Inspired by transfer learning, we train two advanced deep convolutional neural networks (DCNN) with two different large datasets in source domain, respectively. By exploring the complementarity of two distinct DCNNs, deep feature fusion is utilized after feature extraction in target domain. Then, template specific linear SVMs is adopted to enhance the discrimination of framework. Finally, multiple matching scores corresponding different templates are merged as the final results. This simple unified framework exhibits excellent performance on IJB-A dataset. Based on the proposed approach, we have submitted our IJB-A results to National Institute of Standards and Technology (NIST) for official evaluation. Moreover, by introducing new data and advanced neural architecture, our method outperforms the state-of-the-art by a wide margin on IJB-A dataset.
Related Posts
Paper accepted for publication in the IEEE Transactions on Systems, Man, and Cybernetics: Systems
Collaborating with Nanjing University of Science and Technology (NUST), Panasonic R&D Center Singapore co-authored a paper titled “Modular Lightweight Network…
Read morePanasonic R&D Center Singapore Achieves No. 1 Accuracy of Face Recognition on the IJB-C Dataset
Panasonic R&D Center Singapore achieved the Best Accuracy of Face Recognition on the IJB-C dataset under three different protocols, namely 1:1 mixed verification, 1:N mixed identification and 1:1 covariate verification.
Read morePanasonic Tops the IROS 2018 Mobile Manipulation Hackathon Competition 2018 as First-Place Winner
Panasonic R&D Center Singapore teamed up with the Nanyang Technological University to take part in the IROS Mobile Manipulation Hackathon…
Read morePanasonic R&D Center Singapore’s paper accepted for presentation at the IEEE GLOBECOM 2020 and for publication in its proceedings
Panasonic R&D Center Singapore’s paper titled “Big Data Scenarios Simulator for Deep Learning Algorithm Evaluation for Autonomous Vehicles” has been…
Read more