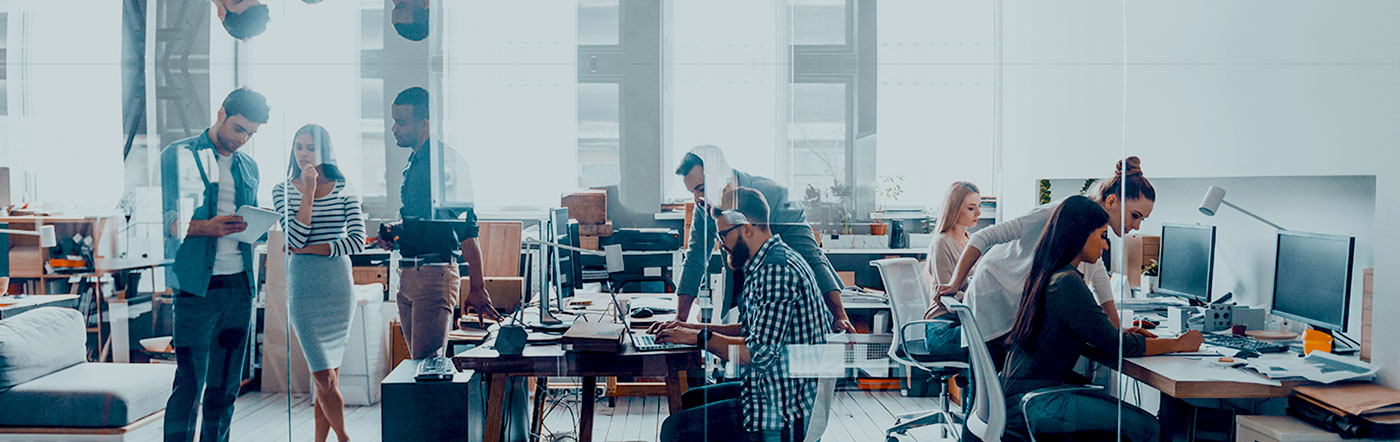
Company News
Look Across Elapse: Disentangled Representation Learning and Photorealistic Cross-Age Face Synthesis for Age-Invariant Face Recognition
|
Jian Zhao, Yu Cheng, Yi Cheng, Yang Yang, Fang Zhao, Jianshu Li, Hengzhu Liu, Shuicheng Yan, Jiashi Feng
The Thirty-Third AAAI Conference on Artificial Intelligence (AAAI-19), 2019.01
Despite the remarkable progress in face recognition related technologies, reliably recognizing faces across ages still remains a big challenge. The appearance of a human face changes substantially over time, resulting in significant intra-class variations. As opposed to current techniques for age-invariant face recognition, which either directly extract age-invariant features for recognition, or first synthesize a face that matches target age before feature extraction, we argue that it is more desirable to perform both tasks jointly so that they can leverage each other. To this end, we propose a deep Age-Invariant Model (AIM) for face recognition in the wild with three distinct novelties. First, AIM presents a novel unified deep architecture jointly performing cross-age face synthesis and recognition in a mutual boosting way. Second, AIM achieves continuous face rejuvenation/aging with remarkable photorealistic and identity-preserving properties, avoiding the requirement of paired data and the true age of testing samples. Third, we develop effective and novel training strategies for end-to-end learning the whole deep architecture, which generates powerful age-invariant face representations explicitly disentangled from the age variation. Moreover, we propose a new large-scale Cross-Age Face Recognition (CAFR) benchmark dataset to facilitate existing efforts and push the frontiers of age-invariant face recognition research. Extensive experiments on both our CAFR and several other cross-age datasets (MORPH, CACD and FG-NET) demonstrate the superiority of the proposed AIM model over the state-of-the-arts. Benchmarking our model on one of the most popular unconstrained face recognition datasets IJB-C additionally verifies the promising generalizability of AIM in recognizing faces in the wild.
Related Posts
Panasonic’s newly launched JZ200 OLED TV powered by Panasonic R&D Center Singapore’s proprietary neural network named Lightweight-and-Versatile Network (LVNet)
Panasonic has announced the launch of its flagship OLED TV for 2021, that is, the JZ2000, which includes a brand-new…
Read morePanasonic R&D Center Singapore Received the ISCIT 2018 Best Paper Award
Teaming up with King Mongkut’s University of Technology Thonburi (KMUTT) to co-author a paper titled “Image Denoising with Deep Convolutional and Multi-directional LSTM Networks under Poisson Noise Environments”, Panasonic R&D Center Singapore (PRDCSG) received the Best Paper Award at ISCIT 2018 held in Bangkok on 26-29 September 2018.
Read moreThe ITS Summit Singapore 2017 @Marina Bay Sands
Panasonic R&D Center Singapore partook in a 2-day Intelligent Transportation Society (ITS) Summit Singapore 2017, which attracted more than 300 delegates, industry partners and speakers from the Asia Pacific countries. At this summit, we showcased our initiatives in autonomous automotive technologies and solutions as well as our efforts to accelerate Singapore’s Autonomous Vehicle landscape.
Read morePanasonic Tops the IROS 2018 Mobile Manipulation Hackathon Competition 2018 as First-Place Winner
Panasonic R&D Center Singapore teamed up with the Nanyang Technological University to take part in the IROS Mobile Manipulation Hackathon…
Read more